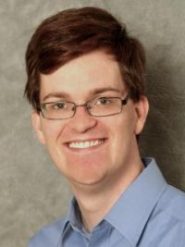
Title: How to Make Causal Inferences Using Texts
Abstract: Texts are increasingly used to make causal inferences: either with the document serving as the treatment or the outcome. We introduce a new conceptual framework to understand all text-based causal inferences, demonstrate fundamental problems that arise when using manual or computational approaches applied to text for causal inference, and provide solutions to the problems we raise. We demonstrate that all text-based causal inferences depend upon a latent representation of the text and we provide a framework to learn the latent representation.
Estimating this latent representation, however, creates new risks: we may unintentionally create a dependency across observations or create opportunities to fish for large effects. To address these risks, we introduce a train/test split framework and apply it to estimate causal effects from an experiment on immigration attitudes and a study on bureaucratic responsiveness. Our work provides a rigorous foundation for text-based causal inferences, connecting two previously disparate literatures. (Joint Work with Egami, Fong, Grimmer and Roberts)
Co-sponsored with the Center for Social Statistics