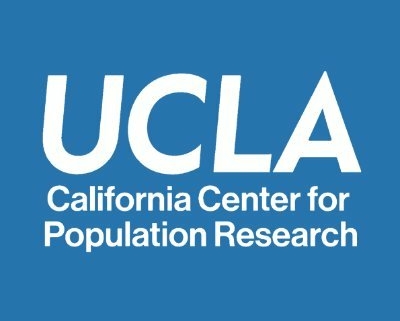
Differences-in-differences Mini-conference
May 24, 2023
UCLA, California Center for Population Research
9-11:30am Speakers hold for meetings
12-1:30pm [CCPR seminar slot] Panel discussion: What’s new with differences-in-differences?
Andrew Goodman-Bacon (Minneapolis Federal Reserve Bank), Alyssa Bilinski (Brown), Jon Roth (Brown), Pedro Sant’Anna (Vanderbilt), Jeff Wooldridge (MSU)
SHORT LUNCH BREAK & ROOM SET UP
2:15-3:00pm Andrew Goodman-Bacon (Minneapolis Federal Reserve Bank), Pedro Sant’Anna (Vanderbilt)
“Difference-in-Differences with a Continuous Treatments”
This paper analyzes difference-in-differences setups with a continuous treatment. We show that treatment effect on the treated-type parameters can be identified under a generalized parallel trends assumption that is similar to the binary treatment setup. However, interpreting differences in these parameters across different values of the treatment can be particularly challenging due to treatment effect heterogeneity. We discuss alternative, typically stronger, assumptions that alleviate these challenges. We also provide a variety of treatment effect decomposition results, highlighting that parameters associated with popular two-way fixed-effect specifications can be hard to interpret, even when there are only two time periods. We introduce alternative estimation strategies that do not suffer from these drawbacks. Our results also cover cases where (i) there is no available untreated comparison group and (ii) there are multiple periods and variation in treatment timing, which are both common in empirical work.
3:00-3:45pm Alyssa Bilinski (Brown)
“Parallel Trends in an Unparalleled Pandemic: Difference-in-Differences for Infectious Disease Policy Evaluation”
Over the course of the COVID-19 pandemic, researchers have extensively studied the impact of public health interventions, such as stay-at-home orders and mask policies, on disease incidence and mortality. Many policy evaluations employ a difference-in-differences (DiD) design, which assumes that treatment and non-experimental comparison groups would have moved in parallel in expectation, absent the intervention (the “parallel trends assumption”). While researchers have used different specifications to capture potential non-linearities, the plausibility of these specifications in the context of dynamic infection transmission is less well-understood. Our work bridges this gap by formalizing epidemiological assumptions required for different DiD specifications, positing an underlying susceptible, infectious, recovered (SIR) model. We first explore common DiD specifications, demonstrating that these often imply strict epidemiological assumptions. For example, DiD modeling raw cases or deaths as an outcome will be biased unless treatment and comparison groups have the same initial conditions, susceptible fraction, and transmission rate (“force of infection”); using a log transformation allows for different initial conditions, but requires equal transmission rates and and susceptible fractions among groups. Furthermore, even if estimates are unbiased, both specifications are often highly anti-conservative under standard error assumptions of a stochastic agent-based SIR model. We then present more robust alternatives, including modeling log difference as the primary outcome and modeling the causal effect of an intervention on the effective reproduction number, rather than cases or deaths. We demonstrate the implications of this work reanalyzing prior work on school mask policies.
3:45pm Coffee break
4-4:45pm Jeff Woodridge (MSU)
“Estimating Distributional Treatment Effects with Staggered Interventions for Panel Data”
I propose simple, parametric approaches for estimating distributions of potential outcomes in a staggered difference-in-differences setting. The approach relies on versions of no anticipation and parallel trends assumptions. Estimators include imputation estimators or pooled maximum likelihood estimation. The approach can be applied to discrete, continuous, and mixed outcomes. A leading application is estimating quantile treatment effects in staggered DiD settings for a continuous outcome.
4:45-5:30pm Jonathan Roth (Brown)
“Log-like? Identified ATEs Defined with Zero-valued Outcomes are (Arbitrarily) Scale-Dependent”
Economists frequently estimate average treatment effects (ATEs) for transformations of the outcome that are well-defined at zero but behave like logpyq when y is large (e.g., logp1 ` yq, arcsinhpyq). We show that these ATEs depend arbitrarily on the units of the outcome, and thus should not be interpreted as percentage effects. In line with this result, we find that estimated treatment effects for arcsinh-transformed outcomes published in the American Economic Review change substantially when we multiply the units of the outcome by 100 (e.g., convert dollars to cents). To help delineate alternative approaches, we prove that when the outcome can equal zero, there is no average treatment effect of the form EP rgpY p1q, Y p0qqs that is point-identified and unit-invariant. We conclude by discussing sensible alternative target parameters for settings with zero-valued outcomes that relax at least one of these requirements.